Kumo empowers deep learning in Snowflake Data Cloud through Snowpark Container Services
5 min read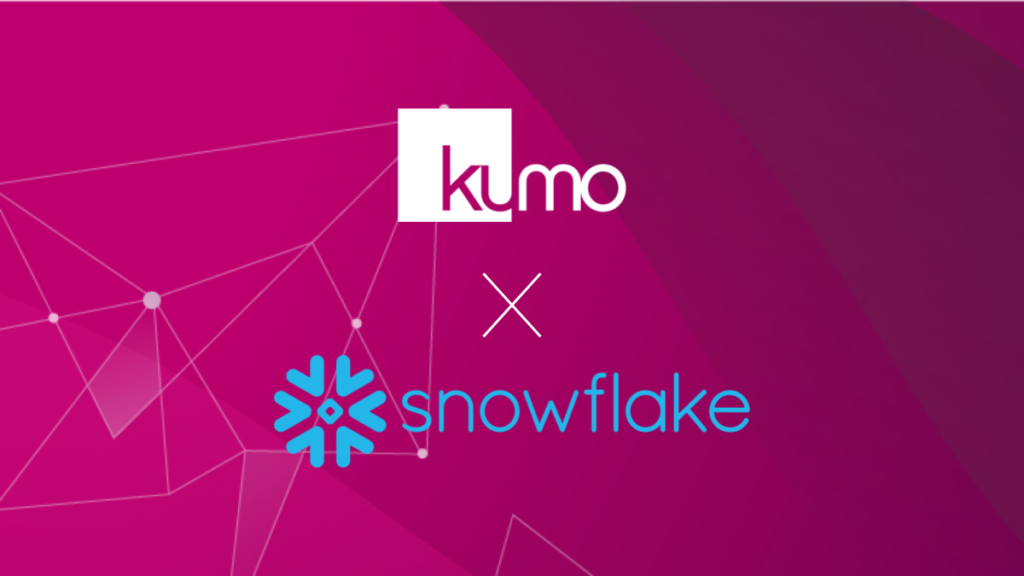
[ad_1]
Join top executives in San Francisco on July 11-12 and learn how business leaders are getting ahead of the generative AI revolution. Learn More
Kumo, a deep learning platform for relational data, announced today at Snowflake Summit 2023 its integration of deep learning capabilities directly into the Snowflake Data Cloud through Snowpark Container Services.
Snowflake’s recently introduced Snowpark Container Services expands the functionality of Snowpark, which allows developers to write code in their preferred language and run that code directly on Snowflake. This Container Services update allows organizations to run third-party software and full-stack applications within their Snowflake accounts.
According to Snowflake, with this integration customers can maximize their data potential by using cutting-edge tools while maintaining data security and eliminating the need for data movement.
Moreover, Snowpark Container Services includes GPU support, which gives data science and machine learning teams a way to accelerate development and bridge the gap between model deployment and consistent data security and governance throughout the AI/ML lifecycle.
Event
Transform 2023
Join us in San Francisco on July 11-12, where top executives will share how they have integrated and optimized AI investments for success and avoided common pitfalls.
Kumo is one of the early adopters of Snowpark Container Services, using the technology to deploy advanced neural networks for enterprises.
Kumo’s predictive AI platform employs graph neural network (GNN) technology, empowering developers, data scientists, analysts and business owners to create and implement highly accurate predictions in production.
Graph neural networks and AI
Traditional machine learning requires extracting data from a data warehouse or lake, followed by manual feature development and tuning. The new integration, now available in private preview, lets joint users operate directly on raw Snowflake tables; generate predictions; and store the results as additional tables within Snowflake.
“The new integration will run Kumo’s AI services directly on relational tables over the cloud without the intermediate steps found in traditional machine learning, such as training set generation and feature engineering, by using graph neural network technology,” Vanja Josifovski, co-founder and CEO of Kumo, told VentureBeat.
Josifovski highlighted that users can create and execute a query that offers predictions, mirroring the process of querying past data for analysis, all without the need to export data from their Snowflake environment.
The announcement follows a recent collaboration between Nvidia and Snowflake that allows customers to customize their generative AI models through the cloud to suit their specific enterprise requirements.
The integration enables organizations to develop generative AI applications using their proprietary data within Snowflake’s Data Cloud environment, eliminating the need to transfer data externally.
Facilitating deep learning-based predictive analytics over the cloud
According to Kumo’s Josifovski, Snowpark Container Services will allow customers to directly utilize Kumo’s predictive AI service within Snowflake for conducting graph learning predictions on their enterprise data.
(Come learn more about data and AI in the enterprise at VB Transform on July 11 & 12 in San Francisco, our networking event for enterprise technology decision makers.)
“An age-old question regarding machine learning and data warehousing has been around where the ML processing runs. By changing the paradigm to run the ML processing in the Snowflake Data Cloud, our companies allow users to expand the use of machine learning and predictions to everyone who has access to the Data Cloud,” Josifovski told VentureBeat. “This is done under a single security program which is much more simplified than operating under multiple security programs.”
Modern AI methods heavily depend on linear algebra calculations, which are highly compatible with GPU processing. Previously, to utilize GPUs, Kumo had to extract the data from the customer’s account and process it externally. With this integration, all data processing occurs directly within the customer’s Snowflake account, including GPU processing.
“The approach of not needing a training set and feature engineering shortens the AI/ML lifecycle significantly,” he added. “We aim to relieve data scientists from repetitive and tedious tasks, to focus on higher-level tasks of defining the right predictive task, evaluating the results and finding the best way to obtain business value from the predictions.”
The company introduced a distinctive feature through this offering: deep learning-driven relational data GNNs.
These deep learning-driven GNNs can learn from the graph and associated attributes, which are determined by non-key columns of the data. Once a graph is constructed, multiple AI/ML tasks can be efficiently trained on the same graph without creating separate training sets or numerous engineered features.
Kumo also offers a scalable and innovative autoML algorithm that alleviates the burdensome process of hyperparameter tuning.
“While GNNs are very effective for a wide range of predictive problems, they are also hard to implement, scale and make efficient. Kumo’s AI platform eliminates the need for graph creation, which requires familiarity with GNNs and optimization task creation. To specify the AI/ML task, Kumo has implemented a predictive query language,” said Josifovski.
Streamlining predictive analytics for citizen developers
Josifovski says that predictive AI/ML currently requires highly skilled specialists with narrow expertise. The lifecycle involves experimenting with features, necessitating substantial infrastructure support for training and inference (scoring).
He explained that the objective of the new integration is to offer users a streamlined workflow, irrespective of their proficiency in data science.
They can then easily apply predictive graph learning in diverse business domains like customer acquisition, loyalty, retention, personalization and fraud detection. His company asserts that an entire AI-based analysis can be completed in a few hours.
“Kumo allows users to run queries over the relational data without requiring a deep understanding of AI/ML concepts, while providing control of the training and inference for skilled data scientists,” said Josifovski. “This way, the platform allows a wide range of users to use it, similarly to how data warehouses are used today for analytics.”
Additionally, Kumo highlighted that the native integration with Snowflake facilitates the installation and usage of the product without requiring security and legal privacy reviews. This reduces barriers and significantly shortens the time to achieve value.
The company is confident that this will expedite experimentation and deployment of detailed predictions, enabling and improving practices such as customer acquisition, personalization, entity resolution and other predictive tasks.
“In enterprises, many teams issue SQL queries over a data warehouse to obtain analytics that professionals then consume to chart future actions,” Josifovski told VentureBeat. “Kumo will allow users to obtain actionable predictions in an automated manner, without requiring professional interpretation.”
VentureBeat’s mission is to be a digital town square for technical decision-makers to gain knowledge about transformative enterprise technology and transact. Discover our Briefings.
[ad_2]
Source link